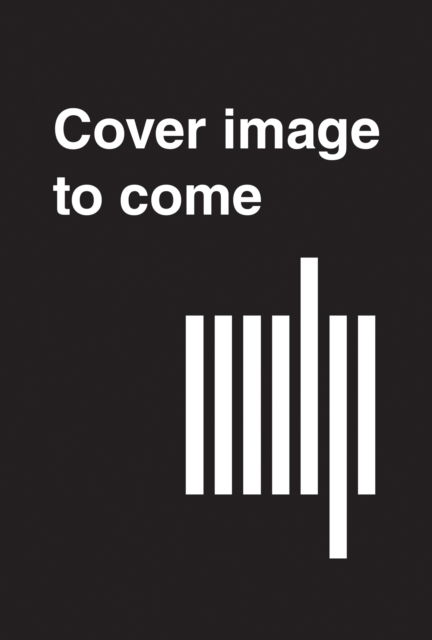
Conte aos seus amigos sobre este item:
Machine Learning from Weak Supervision: An Empirical Risk Minimization Approach - Adaptive Computation and Machine Learning series
Masashi Sugiyama
Machine Learning from Weak Supervision: An Empirical Risk Minimization Approach - Adaptive Computation and Machine Learning series
Masashi Sugiyama
Fundamental theory and practical algorithms of weakly supervised classification, emphasizing an approach based on empirical risk minimization.
Standard machine learning techniques require large amounts of labeled data to work well. When we apply machine learning to problems in the physical world, however, it is extremely difficult to collect such quantities of labeled data. This book presents theory and algorithms for weakly supervised learning, a paradigm of machine learning from weakly labeled data. Emphasizing an approach based on empirical risk minimization and drawing on state-of-the-art research in weakly supervised learning, the book provides both the fundamentals of the field and the advanced mathematical theories underlying them. It can be used as a reference for practitioners and researchers and in the classroom.
The book first mathematically formulates classification problems, defines common notations, and reviews various algorithms for supervised binary and multiclass classification. It then explores problems of binary weakly supervised classification, including positive-unlabeled (PU) classification, positive-negative-unlabeled (PNU) classification, and unlabeled-unlabeled (UU) classification. It then turns to multiclass classification, discussing complementary-label (CL) classification and partial-label (PL) classification. Finally, the book addresses more advanced issues, including a family of correction methods to improve the generalization performance of weakly supervised learning and the problem of class-prior estimation.
320 pages
Mídia | Livros Hardcover Book (Livro com lombada e capa dura) |
Lançado | 23 de agosto de 2022 |
ISBN13 | 9780262047074 |
Editoras | MIT Press Ltd |
Páginas | 320 |
Dimensões | 236 × 183 × 19 mm · 746 g |
Idioma | English |
Mais por Masashi Sugiyama
Ver tudo de Masashi Sugiyama ( por exemplo Hardcover Book e Paperback Book )